.png)
Top 5 books for data analysts and data engineers in 2024
.png)
In the world of data and analytics, upgrading to the constantly changing requirement is not a need but a necessity. With ever-changing technology, techniques, and implementation strategies, most sources of knowledge fail to keep up with the changes, leaving analysts and engineers stranded in time.
However, very few books are perpetual and are the repository of knowledge for data analysts and engineers any day. These books focus on people who play with data analytics, from collating it, cleansing, preparing, and doing transformations. Understanding and building data-intensive applications is crucial for data engineers and analysts, as it encompasses the challenges and intricacies of modern data systems. A key resource in this area is "Designing Data-Intensive Applications" by Martin Kleppmann, which delves into data modeling, storage systems, and distributed systems, highlighting its importance for those in the field.
Some data analysis and data engineering books are listed in no particular order, let’s take a look at them
How to Measure Anything
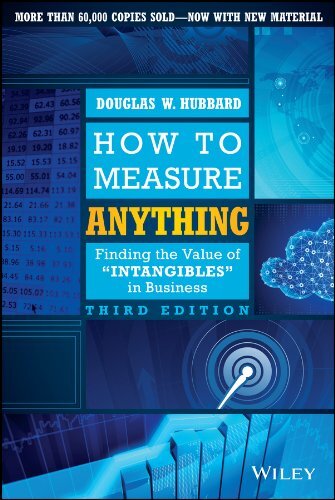
- How to Measure Anything, a 433-page classic written by Douglas W Hubbard emphasizes strictly measuring the data only if you will be doing something useful with it. Well, that summarizes the book. However, the sole purpose of measuring things, which may or may not showcase the exact value but to attain a level of understanding of the value to make an informed decision is what this book is all about.
- We humans are not as good as we think when it comes to predictions, estimating something to its near-accurate value. The way you perceive things would take a different route and the ability to use well-thought-through assumptions and estimates improves significantly as per most readers.
- As this book is all about measurement, it answers most of your questions, what could be the retention rate if there's a change in the brand of any specific product? This book consists of techniques that allow us to approach the business from a different perspective than normal.
Statistics Done Wrong

- Statistics Done Wrong, is a small read with just 176 pages yet power-packed with statistical fallacies, written by Alex Reinhart. A book this crisp, might not serve you the best if you are looking to start from the basics of statistics. The author jumps straight into the practices that could go on to be a disaster.
- This book is not a rant as the title suggests, it's more of the unintentional errors committed by people who work on statistics and this being explained subtly. The book consists of piles of examples with explanations on how wrong statistics can lead to dithering one's business instincts.
- Despite getting all the theories right, the practical implementation of statistics is very hard. Statistics might seem easy on the eye, but that's how loads of research ends up negatively. Statistics done wrong is a guide for what not to do.
Data Science and Big Data Analytics
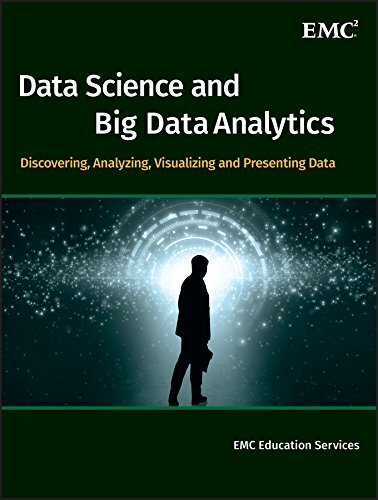
- Data Science and Big Data Analytics is not “just another data science book”, this 432-page delight educates the readers about what Big Data is and how to make the best use of it. The book is a bit vague yet gives great information on all the high-level concepts like randomization, sampling, distribution, sample bias, etc.
- Right from understanding big data and drawing analytics from it to the techniques to analyze structured and unstructured data, machine learning implementations, and data visualizations is what this book speaks about.
- Well-articulated concepts with theoretical and practical support (examples are provided in R) and supplemented with visual interpretations. However, this data science book is for data science beginners, much like a big data analytics book for beginners. As per most readers, it's a preferable handbook to glance at before exams or interviews.
Practical Statistics for Data Scientists

- Practical Statistics for Data Scientists is more like a consolidated record of how the basics of statistics are seen from the data scientist's perspective written by Peter and Andrew Bruce which has a total of 320 pages.
- To make the model easily understandable to programmers, python is used but for non-programmers, a Python course first would make things easy. The book defines the differences between statistics and data science first, the traditional statistics, and how different it is from data science. Not just that, the relation between the two terminologies is also explained.
- The book suggests “50 essential concepts” in its title but as per the readers, it's a lot more than that, the practical examples for resampling, A/B testing, etc, and R code language make this book rich. A must-read data science book for beginners.
An Introduction to Statistical Learning

- An Introduction to Statistical Learning has about 426 pages written by Gareth M. James, Daniela Witten, Trevor Hastie, and Robert Tibshirani on the vastness of data sets and how statistics is an essential tool to derive the best sense from it.
- The topics covered are modeling and prediction techniques along with their applications. In addition to this, linear regression, classification, resampling methods, shrinkage approaches, etc are also explained in-depth.
- As per readers, the best thing about the books is that it facilitates practice at the end of every chapter as every chapter consists of one specific algorithm with R code. This alters perspectives and allows readers to practice these techniques with real-world examples. A book highly recommended for beginners with basic statistics knowledge and who are looking to kick start their career in data science and data analytics.
Frequently Asked Questions FAQs- Top 5 Books for Data Analysts and Data Engineers.
Is data engineering harder than data analysis?
Data engineering is generally considered more technically challenging than data analysis. Data engineers are responsible for building and maintaining the complex data infrastructure and pipelines that power data analysis. This requires a deeper understanding of database management, distributed systems, and software engineering principles.
Which is better, an analyst or an engineer?
There is no simple answer as to whether an analyst or an engineer is "better". Both roles play crucial and complementary parts in the data ecosystem.
Is a data analyst lower than a data engineer?
No, data analyst and data engineer are not necessarily hierarchical roles where one is "lower" than the other. They are distinct functions that require different skill sets and responsibilities. Data analysts and data engineers often work closely together, with each role contributing unique expertise to the data-driven decision-making process.
What is higher than a data analyst?
Roles that are often considered higher than a data analyst include data scientist, business intelligence (BI) manager, and Chief Data Officer (CDO).
What is the highest-paying analyst?
The highest-paying analyst role is often considered to be the financial analyst, particularly in the investment banking and hedge fund industries. Financial analysts who specialize in areas like mergers and acquisitions, equity research, or portfolio management can command some of the highest salaries among all analyst positions.
What book should I read for a data analyst?
For aspiring data analysts, some recommended books include "Storytelling with Data" by Cole Nussbaumer Knaflic, "Data Science for Business" by Foster Provost and Tom Fawcett, and "The Data Warehouse Toolkit" by Ralph Kimball and Margy Ross. These books cover essential skills such as data visualization, business understanding, and data warehousing, which are crucial for data analysts to effectively communicate insights and support decision-making.
Can a data analyst be a data engineer?
Yes, a data analyst can transition to become a data engineer. While the roles have distinct responsibilities, there is significant overlap in the required skills, such as proficiency in data manipulation, SQL, and understanding of data architectures.
Which subject is best for a data engineer?
Aspiring data engineers often have backgrounds in computer science, software engineering, or a related technical field. Subjects such as database management, distributed systems, cloud computing, and programming languages like Python, Java, or Scala are particularly relevant for data engineering roles. Additionally, knowledge of data processing frameworks like Apache Spark, Hadoop, and Kafka can be beneficial for data engineers.
Do data analysts do ETL?
Yes, data analysts are often involved in the Extract, Transform, and Load (ETL) process, which is a critical step in data analysis. Data analysts may help design and implement ETL pipelines, ensure data quality, and transform raw data into a format suitable for analysis. While data engineers typically handle the more complex and scalable aspects of ETL.