
In today's digital world, data has become an invaluable asset for organizations across all industries. However, with the increasing volume, velocity, and variety of enterprise data being generated, managing it effectively has become a daunting task. This is where data governance comes into play.
In this article, we will explore what data governance involves, its benefits, the problems that led to its emergence, key areas within data governance, its framework, and the challenges faced in its implementation.
What is Data Governance?
Data governance refers to the overall management of the availability, integrity, usability, quality, and security of an organization's data assets. It encompasses defining roles and responsibilities related to data management processes while ensuring compliance with relevant regulations and standards. The primary goal of data governance is to establish policies and procedures that enable organizations to make informed decisions based on accurate and reliable data and provide data access to selected business users.
Benefits of Data Governance:
Implementing proper data governance practices can offer the following advantages for organizations:
Improved Data Quality: By implementing proper standards for data entry, storage, and retrieval processes, organizations can ensure consistent and high-quality datasets.
Regulatory Compliance: With increased scrutiny over privacy regulations like GDPR or CCPA, effective data governance helps organizations meet legal obligations regarding customer information protection.
Enhanced Decision-Making: When businesses have access to trustworthy and well-managed data assets through clear governance frameworks, decision-makers can rely on accurate insights for strategic planning.
Increased Efficiency: Well-governed data reduces time spent searching for information or dealing with inconsistencies or errors thereby improving operational efficiency.
Data Governance: Problems That Led to its Emergence
The need for formalized data governance arose due to several significant challenges faced by organizations:
No Single Source of Truth: With data often being stored in various systems or departments, it becomes challenging to integrate and consolidate information for meaningful insights.
Ownership Issues: Without clear ownership and accountability over data assets, inconsistencies arise, leading to decreased trust and hindered decision-making processes.
Low Data Quality: Poorly managed data quality can lead to errors, duplication, and inaccuracies that undermine the effectiveness of business operations.
Compliance Risks: The consequences of non-compliance with regulations have increased significantly, necessitating a structured approach to ensure adherence to relevant legal requirements.
Areas within Data Governance:
Data governance encompasses several key areas that support the effective management of an organization's data assets:
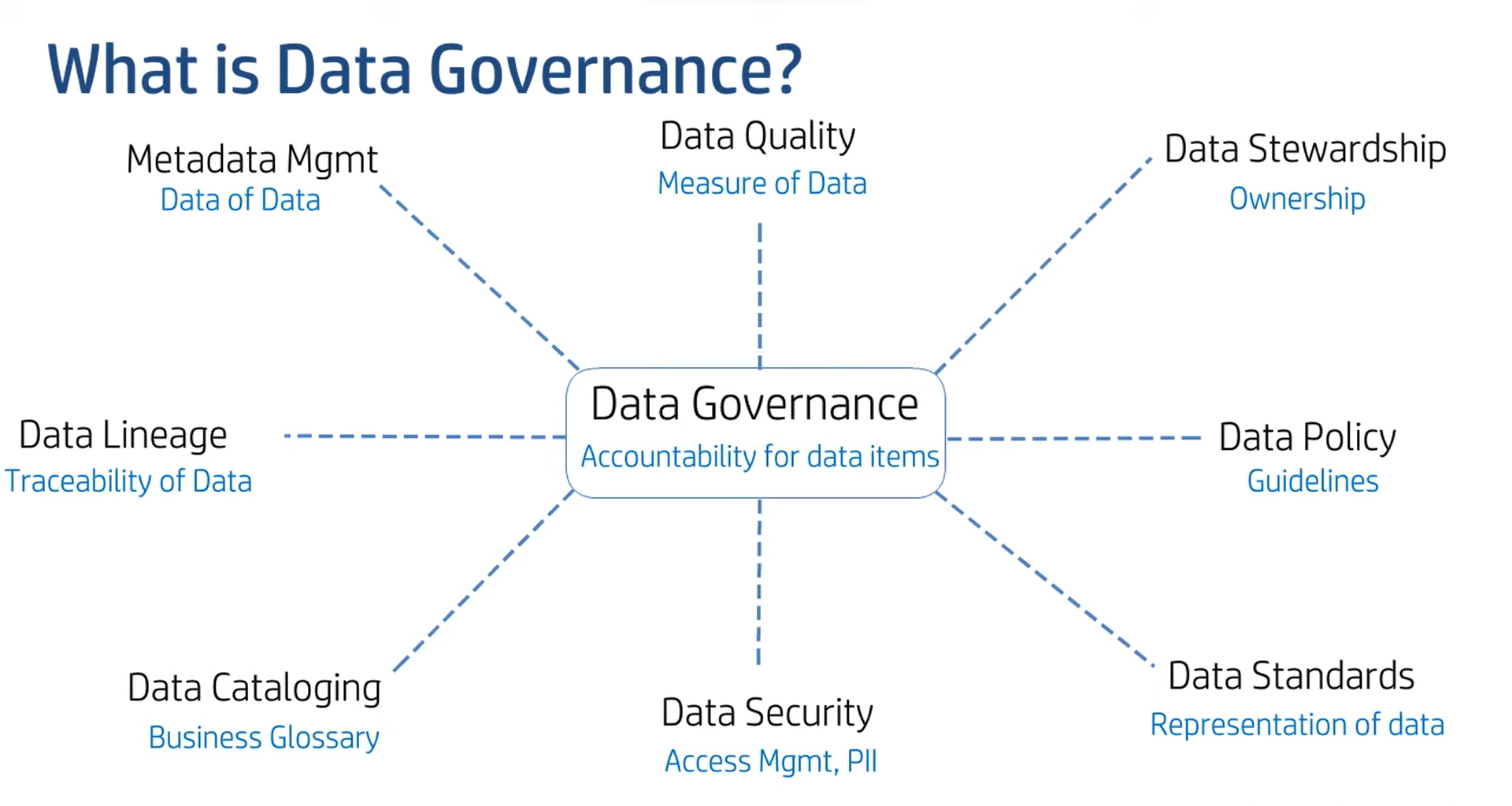
Data Stewardship:
Data stewardship is the process of managing an organization's data assets. It involves ensuring that data is accurate, reliable, and available to authorized individuals when needed. Data stewards are responsible for defining and implementing policies and procedures related to data governance, as well as monitoring adherence to these guidelines. They work closely with various stakeholders, including data owners, users, and IT teams, ensuring proper data lifecycle. Effective data stewardship helps organizations improve data quality, minimize risks associated with data misuse or loss, and enhance decision-making processes.
Data Quality:
Data quality refers to the accuracy and completeness of data within an organization. High-quality data is crucial for making informed business decisions and driving effective operations. Organizations need to establish processes and standards to maintain data quality throughout its lifecycle. This includes validating incoming data at the point of entry, conducting regular audits to identify and rectify errors or inconsistencies, and setting up mechanisms for continuous monitoring of data quality metrics. Investing in data quality initiatives helps organizations avoid costly mistakes caused by inaccurate or unreliable data, improves customer satisfaction through better service delivery, and enables more accurate reporting and analysis.
Data Policy:
A data policy is a set of rules and guidelines that govern how an organization collects, manages, stores, shares, and protects its data assets. These policies define roles and responsibilities related to handling sensitive information, specify access controls based on user permissions or security levels, outline procedures for ensuring compliance with relevant regulations such as data protection regulation GDPR or HIPAA, and address issues such as retention periods or disposal methods for different types of data. By implementing clear and comprehensive data policies, organizations can ensure consistent practices across departments or business units while safeguarding sensitive information from unauthorized access or misuse.
Data Standard:
A data standard refers to a set of conventions that define how customer data should be structured, stored, and exchanged within an organization or across different systems. Data standards provide a common framework for organizing and classifying information, ensuring communication between various applications or databases. They establish rules for naming conventions, data formats, units of measurement, and other technical aspects to ensure consistency and accuracy in data representation. Implementing data standards allows organizations to streamline processes such as data integration or migration, improve data quality by reducing errors or inconsistencies, and facilitate efficient data sharing and collaboration.
Data Security:
Data security involves protecting organizational data from unauthorized access it includes measures such as encryption, access controls, firewalls, intrusion detection systems, and regular security audits to identify vulnerabilities or potential risks. Data security aims to safeguard sensitive information from external threats like hackers or malware attacks as well as internal risks such as employee negligence or intentional misuse. Organizations need to develop robust security policies that outline procedures for handling sensitive data, educate employees about best practices for maintaining data security, and regularly update their security protocols to stay ahead of evolving threats.
Data Catalogs:
Data cataloging is the process of creating a comprehensive inventory of an organization's data assets. It involves categorizing and documenting datasets based on their attributes such as source system, format, owner, purpose, and available metadata. A well-organized data catalog provides users with a clear overview of what data is available within the organization and enables them to quickly search for specific datasets based on their requirements. This helps improve productivity by reducing the time spent searching for relevant data and promotes better decision-making through access to trusted information sources.
Data Lineage:
Data lineage refers to the ability to trace the origins and transformations of a particular piece of data throughout its lifecycle. It tracks how data moves from source systems into different storage locations or analytical platforms while capturing any changes made along the way. Data lineage provides transparency and accountability, allowing organizations to understand the data's reliability and credibility for decision-making or regulatory compliance purposes. It also helps in identifying any potential issues or errors introduced during data integration or transformation processes, enabling timely resolution and ensuring data accuracy.
Metadata Management:
Metadata management involves organizing, documenting, and controlling metadata – that is, information about data characteristics such as its structure, format, meaning, relationships, and context. Effective meta-data management practices enable users to easily find and understand relevant data assets within an organization. Properly managed meta-data improves data discovery, promotes data reuse, enhances collaboration between different teams or departments, and supports effective analysis and reporting by providing context to the underlying data.
Data Governance Framework :

People:
In the data governance framework, people play a crucial role in ensuring effective data management and its protection. This includes individuals such as data stewards, who are responsible for overseeing the quality and integrity of data within an organization.
Data stewards work closely with other stakeholders, such as IT professionals and business users, to define policies and procedures for data handling. Additionally, employees across different departments need to be educated about their responsibilities regarding data governance, including how to properly handle and protect sensitive information.
Regular training sessions can help ensure that all personnel understand the importance of data governance and are equipped with the necessary knowledge and skills to comply with established guidelines.
Process:
The process aspect of a data governance framework focuses on establishing clear guidelines and procedures for managing data throughout its lifecycle. This includes defining standards for collecting, storing, accessing, and sharing data within an organization. Processes should also address issues such as data quality management, metadata management, and data classification.
A well-defined process ensures consistency in how data is handled across different systems and departments. It also enables organizations to effectively respond to compliance requirements and regulatory changes by implementing mechanisms for monitoring and auditing data-related activities.
Technology:
Technology plays a critical role in supporting the implementation of a robust data governance framework. Organizations need appropriate tools and technologies to facilitate efficient storage, retrieval, analysis, and sharing of data while maintaining security and privacy measures. This may include deploying database management systems (DBMS), enterprise resource planning (ERP) software, customer relationship management (CRM) platforms, or other specialized applications tailored to specific industries or sectors. Additionally, technology solutions such as master data management (MDM) systems can help maintain consistent and accurate records across multiple databases or systems.
The use of automation data governance tools can also streamline processes related to metadata management, access control, or monitoring of data usage patterns. Ultimately, selecting the right technology infrastructure is crucial for successfully implementing a comprehensive data governance framework.
Data Governance Best Practices :
Implementing a comprehensive data governance framework is crucial for successful adoption within an organization:
Define Objectives:
Clearly articulate the data governance program's purpose, goals, and expected outcomes aligned with organizational objectives.
Establish Policies:
Develop policies governing all aspects of data management while considering regulatory requirements specific to the industry.
Assign Roles & Responsibilities:
Identify accountable individuals responsible for stewardship activities such as monitoring compliance, enforcing policies, and ensuring data quality.
Create Data Governance Programs and Committees:
Establish cross-functional committees to facilitate decision-making and review the effectiveness of data governance initiatives.
Implement Controls & Processes:
Define processes for data collection, storage, access, sharing, retention, and disposal while enforcing appropriate controls at each stage.
Problem Faced in Data Governance Challenges:
Implementing a robust data governance program is not without its challenges, some of the common challenges faced by business users are mentioned below:
Resistance from employees: Organizations may face resistance from employees who view data governance as an additional burden or hindrance to their existing workflows.
Lack of Resources: Insufficient budget allocation, limited skilled personnel, and inadequate technology infrastructure can impede effective implementation.
Complexity & Scalability: With large volumes of diverse data sources and evolving regulatory requirements, creating a scalable framework that adapts to changing needs becomes complex.
Data Ownership & Collaboration: Determining ownership rights over datasets can be challenging in organizations with decentralized structures, leading to conflicts regarding responsibility and accountability.
Sprinkle Data To The Rescue:
Implementing a robust data governance strategy is crucial for organizations aiming to harness the full potential of their data assets effectively. Sprinkle Data is an excellent solution for addressing these challenges due to its comprehensive features such as:
Monitoring Workflows: It enables easy policy creation, enforcement, and monitoring of compliance levels through automated workflows within the tool.
Role-Based Access Control: It ensures appropriate access rights based on user roles while maintaining security measures aligned with organizational policies.
Workflow Automation: It streamlines approval processes related to data requests or changes by automating workflows within the tool.
Reporting & Data Analytics: It generates comprehensive reports on key metrics and even identifies areas for improvement.
By embracing Sprinkle Data’s capabilities within your organization's overall data governance strategy, you can overcome challenges and achieve efficient management of your data assets, resulting in enhanced decision-making, improved compliance, and increased operational efficiency.
Frequently Asked Questions FAQs - What is data governance?
What are examples of data governance?
Examples of data governance include creating and implementing policies for data quality, privacy, security, and compliance, establishing a data stewardship program, conducting regular data audits, and defining roles and responsibilities for managing data.
What are the benefits of Data governance?
The benefits of data governance include improved data quality, increased trust in the data, better decision-making based on accurate and consistent information, enhanced regulatory compliance, reduced risks associated with data breaches or misuse, and optimized use of resources by eliminating redundant or duplicate data.
What are the main functions of data governance?
The main functions of data governance are to define and enforce data standards and policies, establish processes for capturing, storing, and maintaining data, ensure the availability and accessibility of quality data to users across the organization, resolve conflicts or discrepancies in data definitions or usage, and monitor compliance with regulations and industry best practices.
Why is Data Governance needed?
Data governance is needed because it provides a framework for organizations to effectively manage their growing volume of data assets. It ensures that the right people have access to the right information at the right time while maintaining its integrity and security.
What is the core of data governance?
The core of data governance lies in establishing a set of rules and processes that govern how an organization manages its data throughout its lifecycle. This includes defining roles and responsibilities for individuals involved in managing and using the data and setting up policies for collecting, storing, protecting, sharing, and analyzing the data.
What is a data governance framework?
A data governance framework is a structured approach or methodology that guides organizations in designing and implementing effective data governance practices. It typically includes elements like organizational structure, policies and procedures documentation templates, communication plans, measurement mechanisms, technology infrastructure requirements, etc.
What is the difference between data Governance and data management?
Data governance establishes rules around how an organization manages its overall enterprise-wide data assets, including policies, processes, roles, and responsibilities. On the other hand, data management refers to the practices and techniques used to manage specific aspects of data (e.g., storage, integration, modeling) within an organization's overall data governance framework.
What are some data governance tools?
Some popular data governance tools include Sprinkle Data, IBM InfoSphere Information Governance Catalog, Alation Data Catalog, and Talend Data Fabric.
What are data governance principles?
Data governance principles are guiding concepts or guidelines that organizations follow to establish effective data governance practices. These principles may include ensuring accountability for data stewardship, promoting transparency in data management processes, enforcing compliance with regulations and industry standards, fostering collaboration between business and IT stakeholders, and continuously improving data quality and integrity.
What happens without data governance?
Without proper data governance in place, organizations may face challenges such as inconsistent or inaccurate data leading to poor decision-making, difficulty in complying with privacy laws or regulations, increased risk of security breaches or unauthorized access to sensitive information.